Third Workshop on Machine Teaching for Humans (MT4H)
at ECML-PKDD 2025 Conference
Machine teaching, as the inverse problem of machine learning, involves a teacher designing optimal training strategies to guide a learner toward specific goals. In the context of Explainable AI (XAI), this paradigm offers exciting opportunities to enhance the interpretability, usability, and trustworthiness of AI systems. The workshop will address the following critical issues:
- Developing Human-Centric Teaching Paradigms for Explainability: How can optimal teaching strategies help align AI behaviour with human expectations by enabling models to provide clear, context-aware explanations?
- Integration of Machine Teaching and Explainability: What novel algorithms and theoretical foundations can merge machine teaching techniques with explainable AI to improve interpretability in real-world systems?
- Ethics and Robustness: How can machine teaching address challenges like adversarial manipulation (e.g., training-set poisoning) while maintaining robust and fair outcomes?
- Evaluation and Scalability: How do we evaluate the success of machine teaching for XAI across domains and ensure scalability to complex applications?
Machine teaching is an emerging sub-field in AI with immense potential to revolutionise how humans interact with machine learning systems. It has implications for a wide range of applications, for example, in healthcare, finance, and education, from personalised tutoring systems to securing models against adversarial attacks. Combining this field with explainable AI is particularly timely, as it offers a structured way to make AI systems not only more accurate but also more understandable and accountable.
The third edition of Machine Teaching for Humans (MT4H) Workshop will take place as part of the European Conference on Machine Learning and Principles and Practice of Knowledge Discovery in Databases (ECML PKDD 2025) in Porto, Portugal. By fostering cross-disciplinary collaboration, this workshop will inspire fresh perspectives and catalyse research in areas that may not align with the core themes of the ECML conference, positioning machine teaching as a cornerstone for advancing explainable AI.
Previous versions of the workshop were organised in 2024 in Valencia (Spain) and in 2023 in Madeira (Portugal).
Featured Talks
The workshop was pleased to feature presentations by the following speakers.
- TBA
Call for Papers
We are pleased to announce the Workshop on Machine Teaching for Humans (MT4H), to be held as part of the ECML-PKDD 2025 Conference. This workshop aims to bring together researchers and practitioners from academia and industry to explore the intersection of machine teaching and explainable AI (XAI), focusing on methods, applications, and theoretical advancements that enhance AI systems’ interpretability, usability, and trustworthiness.
Topics of Interest
We invite submissions on (but not limited to) the following topics:
- Theoretical foundations and algorithms for machine teaching in XAI
- Human-centric teaching paradigms for AI explainability
- Machine teaching applications in real-world explainable systems (e.g., healthcare, education, finance)
- Robustness and adversarial challenges in machine teaching (e.g., training-set poisoning)
- Evaluation metrics and benchmarks for teaching-driven explainability
- Ethical and fairness considerations in machine teaching for XAI
- Cognitive and psychological perspectives on teaching AI systems
- Case studies demonstrating successful applications of machine teaching for explainability
Submission Format and Guidelines
We welcome the following types of contributions:
- Regular Papers: Full-length papers (6-8 pages, excluding references) presenting novel research, methods, or applications.
- Extended Abstracts: Short papers (2-4 pages) summarising ongoing work, preliminary results, or visionary ideas.
- Oral-Only Presentations: Authors of recently published or submitted work relevant to the workshop themes are invited to present their contributions orally (no new paper submission required).
Accepted submissions will be included in the joint workshop proceedings published by Springer and made available to attendees. Authors of extended abstracts and oral-only presentations may also submit revised versions to other venues.
Submissions must follow the ECML 2025 formatting guidelines and should be submitted as a PDF via the workshop’s CMT submission platform. Submissions will be reviewed by the workshop program committee based on originality, relevance, quality, and clarity.
Important Dates
- Submission Deadline: June 14, 2025
- Notification of Acceptance: July 17, 2025
- Workshop Date: September 15 or September 19, 2025
Workshop Format And Tentative Schedule
The MT4H workshop will be a half-day program, including a 30-minute coffee break. The structure is designed to balance formal presentations, interactive discussions, and networking opportunities, ensuring a comprehensive and engaging experience for participants.
Tentative schedule: TBA.
Contact
For questions or further information, please contact the workshop organisers at cferri@dsic.upv.es.
We look forward to your contributions and to an engaging workshop on advancing the frontiers of machine teaching and explainable AI.
Workshop Chairs
- César Ferri (Technical University of Valencia, cferri@dsic.upv.es)
- Jan Arne Telle (University of Bergen, Jan.Arne.Telle@uib.no)
- Darío Garigliotti (University of Bergen, Dario.Garigliotti@uib.no)
- José Hernández Orallo (Technical University of Valencia, jorallo@dsic.upv.es)
- Sebastian Lapuschkin (Fraunhofer HHI, sebastian.lapuschkin@hhi.fraunhofer.de)
- Ute Schmid (University of Bamberg, ute.schmid@uni-bamberg.de)
- Patrick Shafto (Rutgers University, patrick.shafto@rutgers.edu)
- Adish Singla (Max Planck Institute for Software Systems, Germany, adishs@mpi-sws.org)
Program Committee members
- TBA
“Machine Teaching for Explainable AI” is a joint project between the Department of Informatics at the University of Bergen, the Valencian Research Institute for AI (VRAIN) and industrial partners Equinor and Eviny. The project is financed by the Norwegian Research Council.
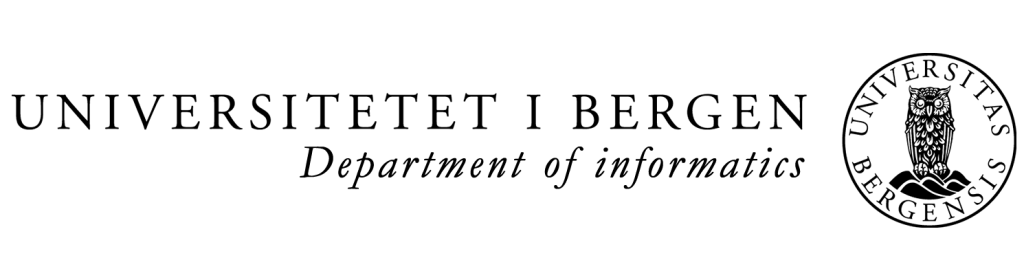